In a recent study published in Molecular Systems Biology, researchers demonstrated that advances in modeling protein-ligand interactions using machine learning-based approaches are needed to exploit AlphaFold2 for antibiotic discovery better.
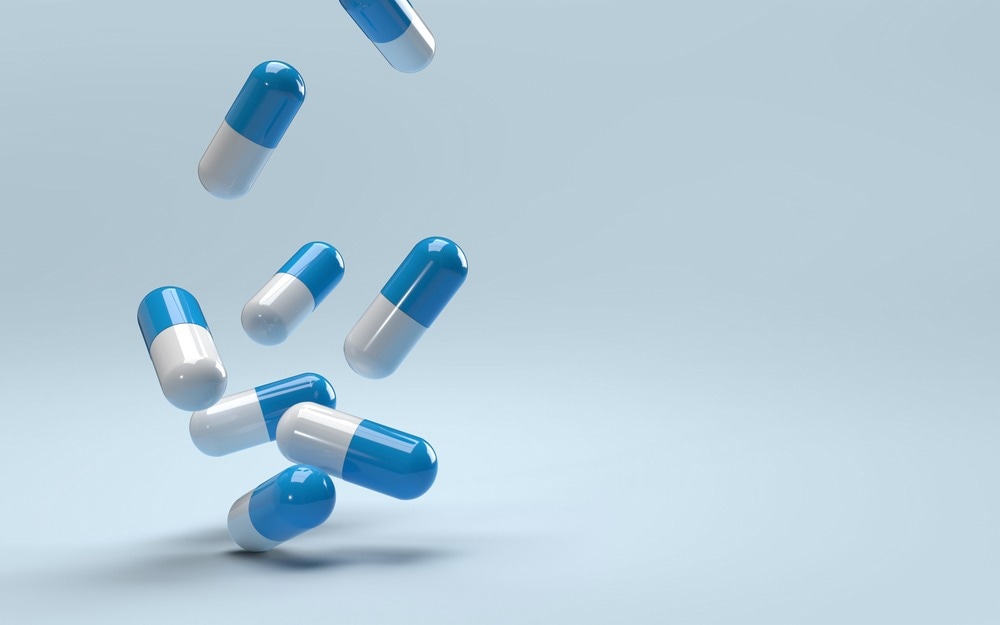
Background
A major challenge in drug discovery is the identification of drug-target interactions. Researchers have deployed several approaches to address this issue, including biochemical assays, genetic interactions, and molecular docking. However, only molecular docking has proven useful for identifying protein-ligand interactions and the mechanism(s) of action of a drug.
Although versatile, docking requires prior knowledge of the protein structures. The number and quality of target protein structures further restrict its application to drug-target identification.
About the study
In the present study, researchers used the recent release of the AlphaFold2 database of protein structure predictions to enable reverse docking approaches that span Escherichia coli's (E.coli) essential proteome, allowing for the extensive prediction of binding targets of antibiotics. These experiments could help benchmark the performance of the modeling platform and divulge the prediction accuracy of AlphaFold2-enabled molecular docking simulations.
The predicted protein-ligand interactions between antibiotics and essential proteins could be experimentally interrogated partly using biochemical assays that measure enzymatic activity, with binding interactions supported by enzymatic inhibition.
The researchers performed high-throughput screens comprising 39,128 compounds of growth inhibition against wild-type E. coli. These compounds were natural materials, antibiotics, and structurally varied molecules with molecular weights between 40 Daltons (Da) and 4,200 Da. All compounds that inhibited relative growth by 80% were considered active, and each active compound was computationally docked with 296 AlphaFold2-predicted E. coli essential proteome.
As a control, a subset of the inactive compounds was docked in the same way. The researchers used AutoDock Vina, a widely used and benchmarked open-source docking program, to dock all 218 active compounds against the 296 AlphaFold2-predicted essential proteome. These simulations predicted both specific and widespread protein-ligand interactions and protein promiscuity. Finally, the researchers rescored predictions with four machine learning-based scoring functions (SFs), viz., RF-Score, RF-Score-VS, protein-ligan extended connectivity (PLEC) score, and neural network (NN)-Score.
Study findings
Genetics & Genomics eBook
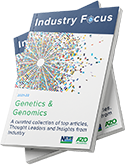
Compilation of the top interviews, articles, and news in the last year.
Download a copy today
In total, 218 antibacterial compounds were active against E. coli, and around 80% were antibiotics of the β-lactam, aminoglycoside, tetracycline, quinolone, and polyketide structural classes. The residual active compounds were comprised of toxins and antineoplastic compounds. The study also identified an additional set of compounds whose antibacterial properties against E. coli have not been documented previously.
Likewise, the study analysis predicted the binding pose and binding affinity of 64,528 protein-ligand pairs. Another 100 inactive compounds selected randomly resulted in binding pose and affinity predictions for 29,600 protein-ligand pairs via analogous docking simulations. Further, the researchers measured the enzymatic activity of several E. coli proteins are involved in deoxyribonucleic acid (DNA) replication, transcription, and cell wall synthesis.
Intriguingly, multiple protein molecules enzymatically inhibited each identified antibacterial compound, confirming extensive promiscuity. This phenomenon also enabled benchmarking of model performance at a statistically significant scale.
The researchers extensively compared experimental data of protein-ligand interactions with in silico predictions to demonstrate that depending on the binding affinity threshold used, this modeling approach had a prediction accuracy between 41% and 73%. Regardless of the binding affinity threshold, the area under the receiver operating characteristic curve (auROC) across the essential proteins averaged 0.48.
Notably, the model performance remained similar even when experimentally determined protein structures were deployed by the researchers. Provided a random model corresponds to an auROC of 0.5, these results indicated that molecular docking simulations displayed a frail performance.
The authors noted a profound improvement in model performance, as measured by the auROC, with RF-Score, RF-Score-VS, and NN-Score. Conversely, the model performance did not improve when DOCK6.9 was employed, and rescoring was performed using the PLEC score. Moreover, consensus models comprising several machine learning-based SFs improved the ratio of the true-positive rate to the false-positive rate and prediction accuracy.
Conclusions
The current study demonstrated that using AlphaFold2 for drug-target prediction is a promising method but is still in its nascent stage. Accordingly, realizing its potential for drug discovery will require substantial improvements in modeling protein-ligand interactions. Benchmarking the performance of molecular docking simulations is one of the feasible ways to improve prediction accuracy; however, it requires the concomitant use of machine learning-based approaches. Overall, the study findings could inform the appropriate use of AlphaFold2 in drug discovery.
- Benchmarking AlphaFold-enabled molecular docking predictions for antibiotic discovery, Felix Wong, Aarti Krishnan, Erica J Zheng, Hannes Stärk, Abigail L Manson, Ashlee M Earl, Tommi Jaakkola, James J Collins. Molecular Systems Biology. doi: https://doi.org/10.15252/msb.202211081 https://www.embopress.org/doi/full/10.15252/msb.202211081
Posted in: Drug Discovery & Pharmaceuticals | Medical Science News | Medical Research News | Pharmaceutical News
Tags: Antibiotic, binding affinity, Cell, Cell Wall, Compound, DNA, Drug Discovery, E. coli, Genetic, Ligand, Machine Learning, Protein, Proteome, Tetracycline, Toxins, Transcription
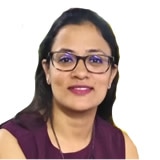
Written by
Neha Mathur
Neha is a digital marketing professional based in Gurugram, India. She has a Master’s degree from the University of Rajasthan with a specialization in Biotechnology in 2008. She has experience in pre-clinical research as part of her research project in The Department of Toxicology at the prestigious Central Drug Research Institute (CDRI), Lucknow, India. She also holds a certification in C++ programming.
Source: Read Full Article